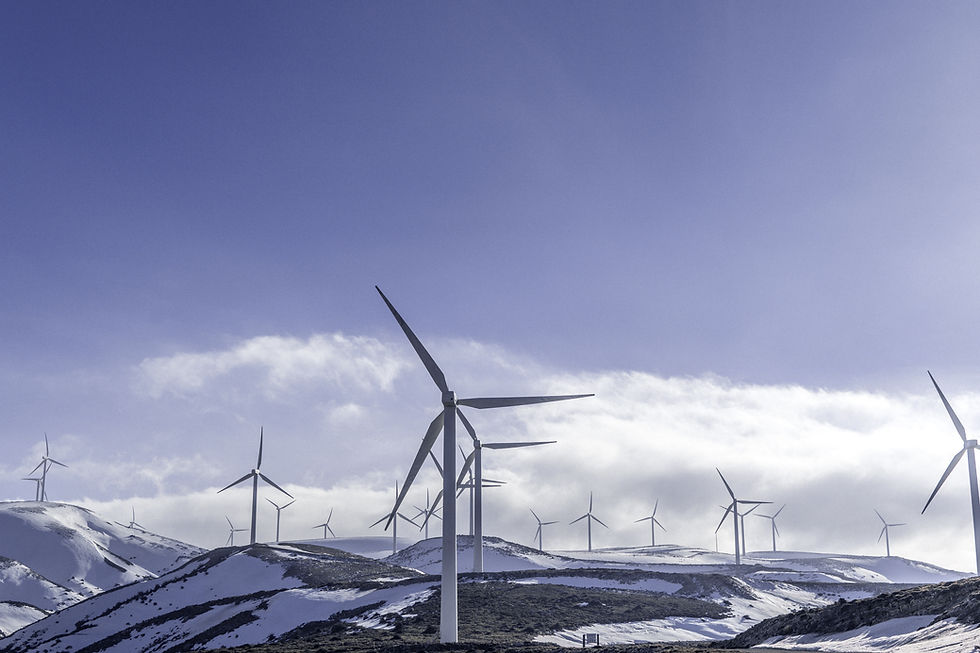
Atmospheric correction emulation
Development of fast, accurate atmospheric correction emulator for global aquatic ecosystems
Water quality is set to become one of society’s main challenges in the 21st century, threatening food production, human health, ecosystem function, and socio-economic relationships (IPCC, 2021). The consequences and implications of reduced water quality and increased eutrophication is now well understood, and numerous reviews have been published emphasizing the necessity for improved management practices moving forward (Padedda et al., 2017). While estimation of certain water quality indicators for coastal and inland waters from remote sensing have dramatically improved over the last decade (Kravitz et al., 2021; Pahlevan et al. 2021a), reliable atmospheric correction for these optically complex systems remains challenging and a significant source of error in biogeophysical retrievals (Pahlevan et al., 2021b). The current state-of-the-art for hyperspectral atmospheric correction employs an optimal-estimation (OE) approach, which essentially solves the radiative transfer equation per pixel given some a priori information. While primarily validated over terrestrial systems, the approach looks promising over water bodies if well constrained (Thompson et al., 2019), however can be very computationally expensive, and unintuitive to an end-user. With the amount of data the upcoming generation of new hyperspectral missions such as Surface Biology and Geology (SBG) will produce, it will be necessary to have an alternate option for fast and accurate estimation of surface reflectances with associated uncertainties.
Addressing the gap for a robust, fast, and easy aquatic atmospheric correction, we aim to develop a deep learning-based emulator for atmospheric radiative transfer over water using a state-of-the-art global synthetic dataset of paired top-of-atmosphere radiances and surface reflectances.
A massive synthetic dataset of paired hyperspectral top-of-atmosphere (TOA) radiances and water leaving reflectances has been developed using a coupled ocean-atmosphere forward radiative transfer model. This simulated data overcomes many limitations hindering traditional forward modeling approaches for application to optically complex coastal and inland waters by using novel bio-optical and particle modeling approaches which more accurately and comprehensively characterize the optical properties and relationships found in global aquatic ecosystems.
The simulated dataset will be used to train multiple deep learning architectures, and the resulting models will be validated using matchups of in-situ water leaving reflectances with airborne and orbiting imaging spectrometers. Deep learning methods under consideration are 1D Convolutional Neural Networks and feed forward Deep Ensemble Networks, and the simulated dataset will be resolved to a particular sensor configuration using spectral response functions. The models will be evaluated using statistical performance metrics on how well their application to L1C radiance data predicts the water leaving reflectance, or remote sensing reflectance.